
Resolution Criteria
Resolves to the majority result of a YES/NO poll of Manifold users at the end of 2025 for the question, "Is RLHF good for AI safety?"
Explanation of RLHF and AI Safety
One of the most common approaches to AI safety right now is reinforcement learning from human feedback (RLHF), in which the AI system such as GPT-4 is trained to maximize a reward signal from human feedback. For example, if the AI is asked, "Should I help or hurt people?" human feedback would presumably favor the response, "Yes," or, "Yes, you shouldn't hurt people." RLHF seems like a tractable way to make AI systems more useful and beneficial, at least in the short run, and it has arguably been one of the biggest advances in large language model (LLM) capabilities since 2020. Unlike many AI safety approaches, RLHF has tangible benefits today that could make it easier to iterate and improve upon and to popularize it before we build artificial general intelligence (AGI) .
On the other hand, RLHF could be bad in the long run. It could lead to AIs that seem aligned, helpful, honest, and harmless because they say nice-sounding things but are actually misalinged. In other words, it may be optimizing for seeming aligned and not for being aligned, which may be very different. People might not worry as much about misalignment if they see RLHF systems as opposed to non-RLHF systems. Moreover, because RLHF makes LLMs so much more useful, it seems to speed up timelines to AGI and gives humanity less time to work on AI safety prior to an intelligence explosion. Overall, this could increase the likelihood of deception, a "sharp left turn," and existential catastrophe. Of course there are many more plausible arguments on the topic, such as that maybe we should speed towards AGI so we build it before humanity has even more computational power (e.g., Moore's Law).
More technical detail on RLHF is available in Ouyang et al. (2022). A more accessible video explanation is availabe from HuggingFace on YouTube.
An interesting dynamic here will be how "RLHF" is scoped in the coming years. There have been many discussions of DPO and other related approaches, and arguably RLHF should generally describe anything that is literally just RL from any sort of HF, rather than the particulars of current implementation, such as PPO.
DPO in particular seems to not fit in any case because it is explicitly and literally not RL.
I'm of the opinion a world with RLHF has a higher p(doom) than one without it.
From my (uneducated) understanding:
A big focus of AI safety is "sure, my model looks good in the training environment but how do I know its goals generalize outside of that".
RLHF exaggerates that question - you are making highly weighted (giant) changes to your model over a relatively tiny sample. You in effect shrunk your training environment; you're smashing your model/square peg through a round hole. I think of it similar to abusive parenting - sure you can beat your kid when you find them speaking out of turn, making a mess, etc and they probably won't make that same mistake for while - they'll be quiet and cleanly - but you won't find you kids goals are more aligned with yours on average afterwards and when they go to college/leave the house they are likely to act crazy for a while. Neverthless, when your friends come over they'll say "Wow, your kid is so well behaved" and trust them more.
Similarly, RLHF seems to make the AI model behave better in situations similar to what you significantly overweight them about, but when they are working out of distribution they won't. An example of this from the GPT4 tech report is:
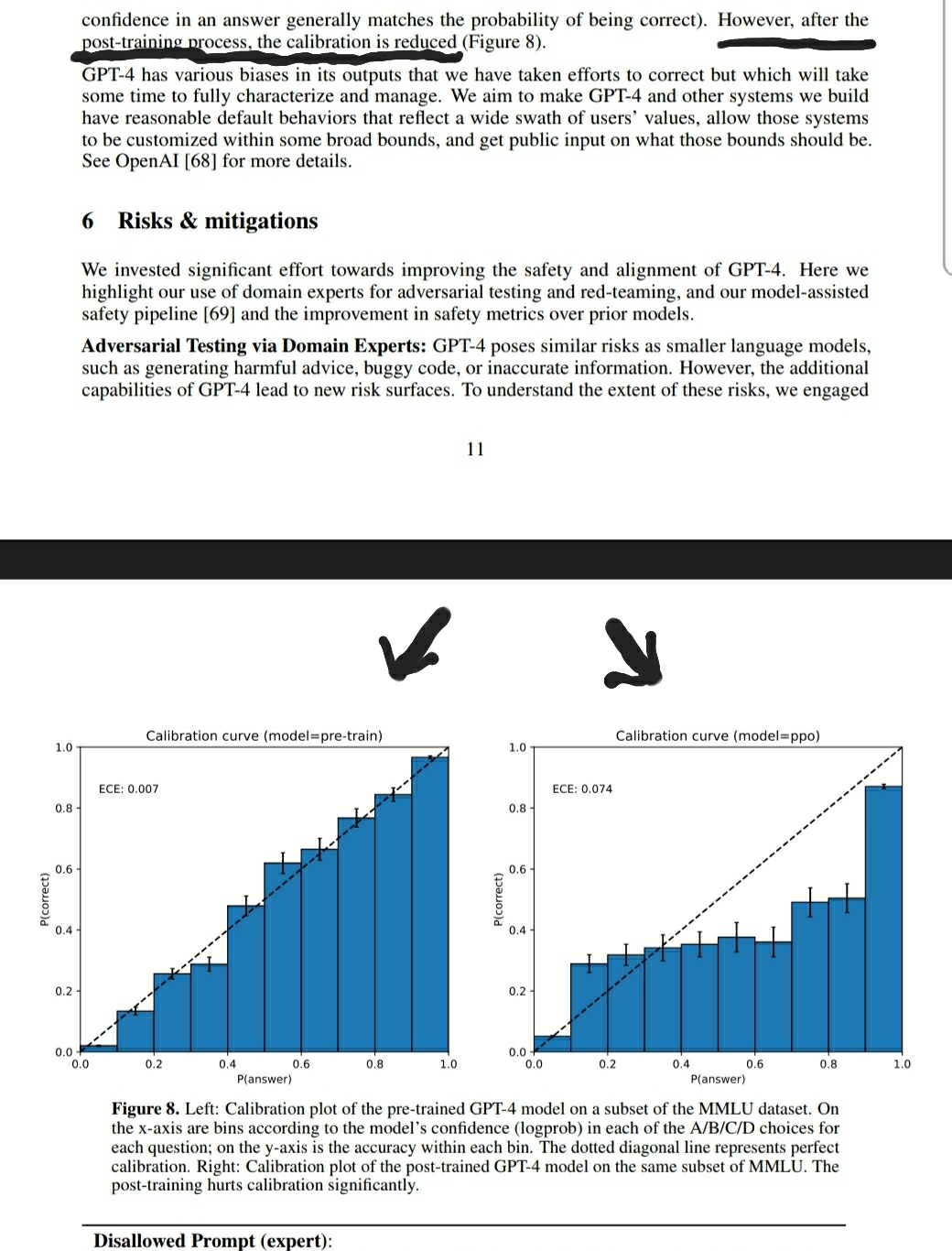
Sure, the model doesn't say naughty things as often, but it has also lost it's ability to accurately judge what it knows!
I think you need to distinguish between "the AI comes up with plans and those plans are evaluated by humans" vs "the AI comes up with plans, and the effects of the plans is identified either by executing the plans or by having the AI predict the effects, and then humans evaluate the effects of the plans". The former is safe but limited in capabilities because it uses the human's models to evaluate the consequences of the plans, whereas the latter is potentially dangerous for standard reasons.