EG "make me a 120 minute Star Trek / Star Wars crossover". It should be more or less comparable to a big-budget studio film, although it doesn't have to pass a full Turing Test as long as it's pretty good. The AI doesn't have to be available to the public, as long as it's confirmed to exist.
@RiskComplex Yeah three more years and you can barely make a 5 second clip.
Ask yourself, as Altman would put it, "if you believe in your heart" that we are 25% closer to full length high-quality movies than we were a year ago.
@DavidBolin Regardless if we're talking about LLMs, GANs or any other architecture the one thing that's clear is that progress is anything but linear. In 2018 machine learning experts thought it would take ~30 years before we had an AI capable of writing a novel. What they didn't take into account is that each progressive increase is larger and takes less time than the previous one. You're making the same mistake.
Sora doesn't have object permanence, no understanding of physics/objects, and there is no proposed approach to create these things. It is a wide open research problem, nobody knows how to even start. This market is ridiculous at 40%. The biggest risk is the market owner just decides that a movie where character's legs blend together still counts as "pretty good" and resolves YES.
there is no proposed approach to create these things
There are at least 2 approaches to get object permanence:
1. Scale is all you need: Sora appears to be about about the same quality/cost as (and by implication the same scale as) open source models like Hunyuan (a 13B parameter model). There is no technological barrier (only cost) preventing us from going >100x bigger (GPT-4 is ~2T parameters). Machine learning runs appear to be doubling in size every 5-6 months, so in 2028 we should expect a Sora-like model (which was trained over a year ago) to be 2**10=1000x more powerful.
2. Models like Google Genie and GameNGen do display object permanence. Most likely, this is because the are trained on long continuous video game runs instead of short clips from the internet.
1. "Scale is all you need" is the mantra of people who don't understand computation. The more parameters you add to your model, the HARDER it is to train it. We are looking for a needle of extremely specific behavior (respecting physics); expanding the model just makes the haystack exponentially larger.
2. You linked to GameNGen, claiming it has object permanence, and literally in the first 5 seconds of the main video at your link, we see failure of object permanence (vanishing barrel). Within the next 20 seconds we see further failures of object understanding (barrels slide across the floor).
Overall, I don't think you understand what "a proposed approach" means. You're just saying: keep training models the same way and hope for the best. Kind of like hoping that mashing the keyboard at random will by chance guess a 50-digit password.
@pietrokc I'm holding No, but just saying - your argument could have been used equally well pre-2020.
@spider Yes, and it would have been correct then as well. State-of-the-art LLMs demonstrably don't have anything close to a "world model", here [1, 2] are just two of many papers published on this topic that I happened to read in the past month or so. Again, training a model to predict the next token and hoping it would figure out the structure of the world from that has always been bananas. Model training is just greedy search! Greedy search doesn't even work for stupidly simple problems from coding interviews!
We're just much better at detecting failures of the world model in video than in text, both because we have evolved much longer to process video-type data than text, and because there are many more chances for errors per second with video.
@pietrokc Sorry- are you trying to deny order-of-magnitude improvements in SOTA world-modeling between GPT-2 era stuff and what we have now? What??
Your actual argument (the parameter space is big, and searching for good models is hard) is fully general, it would apply equally well to million-parameter models as to what we're expecting in the next few years.
@spider Just to make sure we're on the same page, what metric do you have in mind when you refer to "order of magnitude improvements in world-modeling"?
@pietrokc Imo any reasonable definition of "world modeling" is one that's measurable through literally any of the benchmarks about reasoning over and stating true things about the natural world. The first result I found on google has questions of the form:
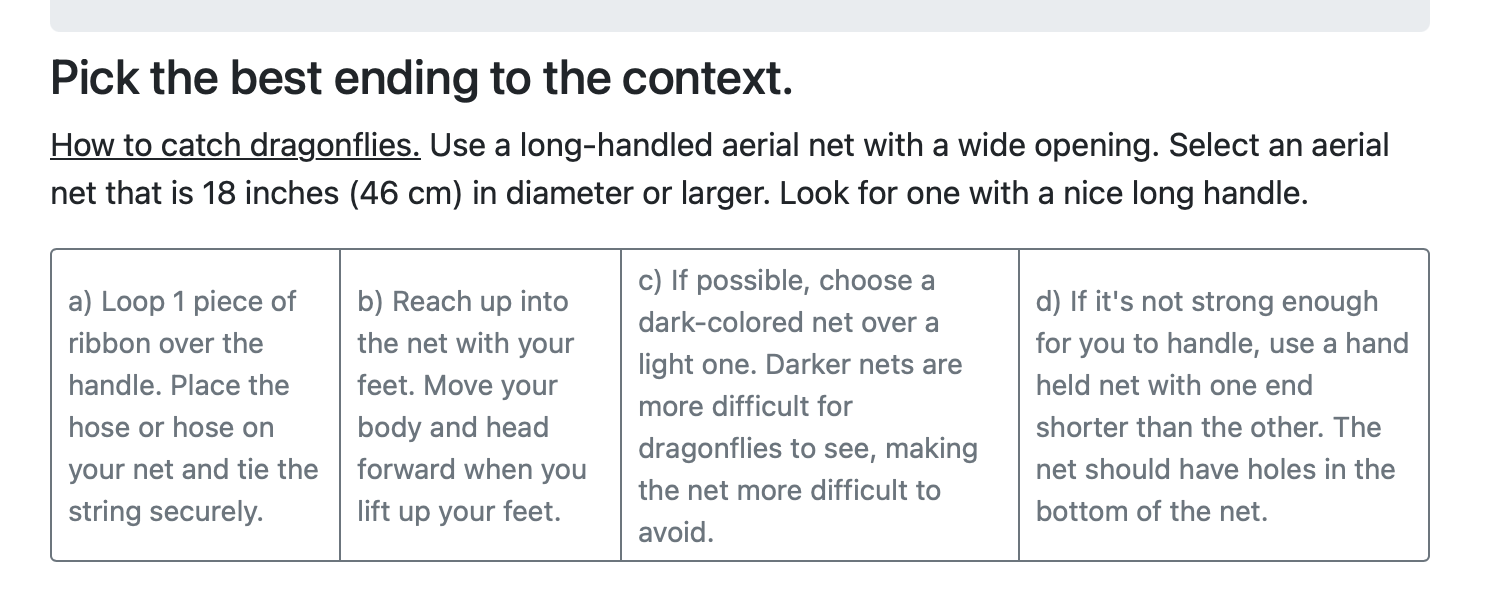
on which 2019 GPT performs at 41.7%, and GPT-4 performs at 95.3%. Converting to odds of getting a question right (the domain in which order-of-magnitude is a measurable thing), that's 0.715 : 1 for the former model and 20.277 : 1 for the later. So, yeah, about a 1.45 order-of-magnitude difference.
It doesn't matter if that's just more memorized facts - any mutable lookup table is a (not very good) world model, provided it does the job of modeling the world.
It also looks like humans are only performing at 95.6% on this dataset, so it might be capping out in terms of measuring power - actual improvement could be greater.
@spider I think there are different types of world modeling. LLMs are greatly improved at handling textual descriptions of the world, but they still struggle with modeling realistic physical objects., which I think is what @pietrokc is describing.
For example, this video is supposed to show a gymnastics floor routine, but it has random arms and legs appearing and disappearing constantly: https://bsky.app/profile/labuzamovies.bsky.social/post/3ld2c4wls322j
@pietrokc "Scale is all you need" is also the mantra of all the people who brought you all the latest foundational models: OpenAI, Google Mind, Anthropic, etc.
@pietrokc "You're just saying: keep training models the same way and hope for the best. Kind of like hoping that mashing the keyboard at random will by chance guess a 50-digit password."
Except the model parameters are not sampled at random but rather arrived at through gradient descent. It would be like guessing a 50-digit password but each time you hit enter you don't try a random string but rather you get closer to the password.
@RiskComplex diffusion models are not the same as LLMs, lol.
But putting that aside, the leap from GPT-2 to o1-pro would not be nearly enough to get from where we are now to generating Hollywood-quality movies from a single prompt!
But regardless, that was FIVE years ago, not three! Even GPT-3 was well over three years ago.
@benshindel You think gradient descent is limited to LLMs? lol How do you think diffusion models, GANs, etc function? lol
@RiskComplex my point is that there's not really any indication that you can simply extrapolate scaling behavior in LLMs to diffusion models
@benshindel You don't need to extrapolate. You can read the dozens of papers on the topic that shows what happens as you scale. e.g., https://www.aimodels.fyi/papers/arxiv/scaling-laws-diffusion-transformers (just the top google result)
@RiskComplex it's not that there are no scaling laws for diffusion, but that scaling BEHAVIOR doesn't extrapolate from LLMs to diffusion models. In LLMs there's been pretty good success with performance improving logarithmically as a function of parameters, but this is not necessarily the case with diffusion models!
@benshindel let me reiterate, the gap between GPT 3.5 and GPT o1-pro (roughly 3 years apart) is nowhere NEAR the gap between the Sora we have now and hollywood-level movies with a single prompt. I mean, even if you look at the context length gap between 3.5 and o1-pro, that will not take you from 20 second clips to 2-hour movies!!!
@benshindel I’m not sure the model itself needs to advance as much as you think for progress to be made.
Consider this scenario: a system like Sora evolves to the point where 1 in 50 videos it generates is movie-worthy. If the movie-generating system includes an agent capable of filtering out videos that violate physics, it could identify and discard the bad ones.
With this approach (or similar methods), the AI doesn’t need to produce high-quality videos consistently. It only needs to generate good ones at a reasonable rate and include a mechanism to evaluate their quality. The limiting factor, then, becomes cost of inference.
Does this sound feasible?
@TiagoChamba okay but someone still has to make such a sophisticated software that can do such things on its own on the basis of a single prompt. Will this be ready for market in 3 years? AI moves fast but it’s not magical!
But putting that aside, I struggle to imagine a world where video generating AI can make 50 movies worth of video where somehow 2% is Hollywood quality and another AI can just parse that and make a movie out of it
@benshindel I'd like to clarify that I'm not arguing either for or against AI actually achieving this. I just wanted to note that an AI that can kind of do the job sometimes may already be enough.
>I struggle to imagine a world where video generating AI can make 50 movies worth of video where somehow 2% is Hollywood quality and another AI can just parse that and make a movie out of it
What part of that do you see as unlikely? I think I'd break the scenario down as follows
AI video generation, through a combination of scaling and maybe some new techniques, that it can sometimes do good clips (following physics, consistency, etc). Not always, just sometimes.
Another AI gets trained to differentiate good videos from bad videos. It may be trained with human judgements of many AI videos.
The generation of suitable videos requires an amount of compute that some company is willing to muster up.
@LoganZoellner Right, I just wanted to lay out the construction of the system, for argument's sake. But it's just GAN lol.
@TiagoChamba I just don't think that AI will be just on this threshold where it can sporadically produce content good enough to be a hollywood-level movie (an incredibly impressive task that would basically remake the world's entertainment industries) and yet somehow mostly produce content inferior to that, such that you need some internal mechanism to differentiate the gold from the slop.
That's like if you told me that aliens have landed on Earth, and that their spaceship is at a tech level of the Apollo space program.
LLMs are currently slightly below top human performance in a few domains. ChatGPT can make decent poems, but nod many Dostoievsky-level texts. Diffussion models generate good art, but not many masterpieces.
I don't think it's implausible that a system gets made that works some of the time. AI progress tends to overcome benchmarks in the most ambiguous, anticlimactic way. At first, a computer was able to play a few chess moves in a specific situation. Then it gets slightly more sophisticated, but is still seen as dumb. When it beats most humans, that's just because it bruteforces the problem! It is just a half-victory! And then, Kasparov loses againt a computer. Now, most chess GMs will readily admit that computer understand the game at a much deeper level than they do.
I don't see how your argument applied to movie production but not these other areas.
@TiagoChamba
if only life were chess…
Ultimately, the generation of an entire movie from a single prompt is a harder problem on like ten different frontiers than getting good at chess.
I mean, Deep Blue beat Kasparov in 1997, well before AI. And at the time it basically was just brute force search. I don’t think that’s particularly useful as an analogy for this problem. And I don’t think that it’s IMPOSSIBLE for a computer to generate a (good) movie like this. I just think there’s very low probability it happens within 3 years. 10 years seems more plausible, though still not certain by any means.
@benshindel That main different between whether a task is 'easy' vs 'hard' for machine learning is compute power needed for training. Chess < Language < Video. Chess was 'easy' because the compute required was trivial. Large language models on the other hand required OOM more compute to work, but fundamentally, for basic large language models, we had the solution decades ago. Things like transformers lowered the amount of compute needed and made LLMs possible now, but even without transformers we would have the ability to make ChatGPT-4o within a decade if compute increased at a linear rate.
We are in the same place with video. We know how to do it. We just lack the compute. So the answer to this question will resolve to YES if one of two things happens: we have a breakthrough that lowers the compute needed OR we develop more compute.
Whether or not we will have a breakthrough that lowers the amount of compute is unknown. But the question of whether or not we will have enough compute is pretty obvious: Since ChatGPT, the rate at which global compute power is being increased at this very moment is incomprehensible. We are literally at the point where small nuclear reactors are being set up to power a single datacenter. We currently have the compute required to make a ~5s video in a few minutes. This means we have enough to make a 24 minutes of video in a day. Of course there are things like consistency issues, artifacts, hallucinations, etc, etc.
But if you think we won't have enough compute in 3 years for a movie studio (that often takes months to film a movie) to spend a few months of compute to make a ~1.5hr video, you're simply wrong, and not wrong by a little, but by orders of magnitude.
@benshindel I'm not arguing about how far away movie generation is. I just wanted to note that there may be a stretch of time where AI can't quite do a movie in 1 try, but the market could still resolve.
@pietrokc Scott Alexander's estimate of this probability -- when he made this prediction -- was 2% or so.
He is not going to bend over backwards to try to make it resolve yes. It may even resolve NO if you really do have AI movies by then, if he doesn't like the quality.